At King, we bring moments of magic to hundreds of millions of people, every single day.
We’re continuously experimenting, learning and adapting to shape the industry in ways yet
to be imagined. And we’re not afraid to have fun along the way. Together, we’ll create new experiences that raise the bar, delight millions of people and redefine the world of games.
Are you ready to join us in Making the World Playful?
to be imagined. And we’re not afraid to have fun along the way. Together, we’ll create new experiences that raise the bar, delight millions of people and redefine the world of games.
Are you ready to join us in Making the World Playful?
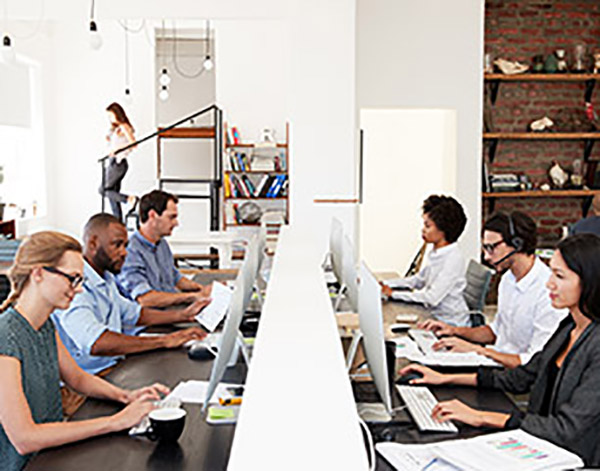
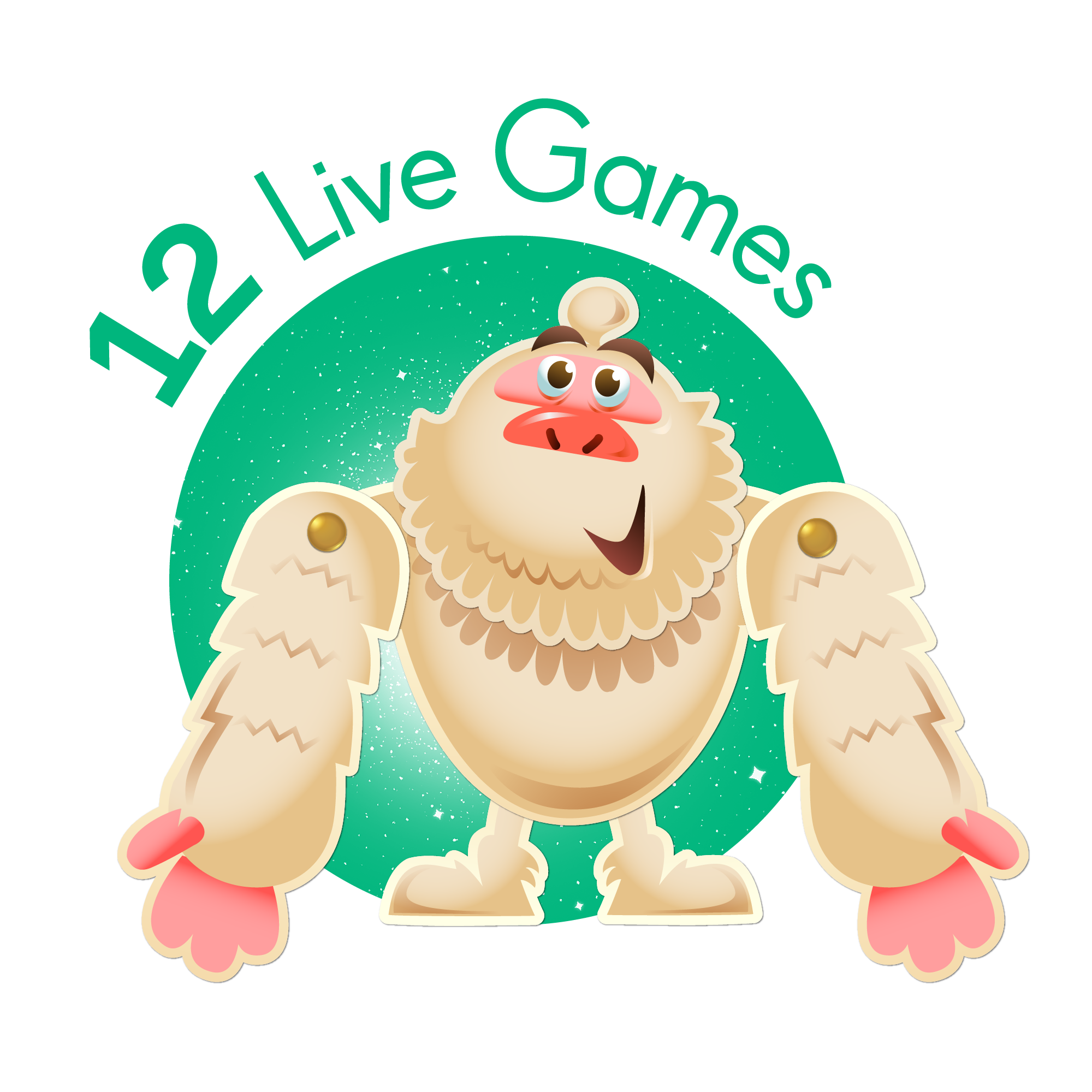
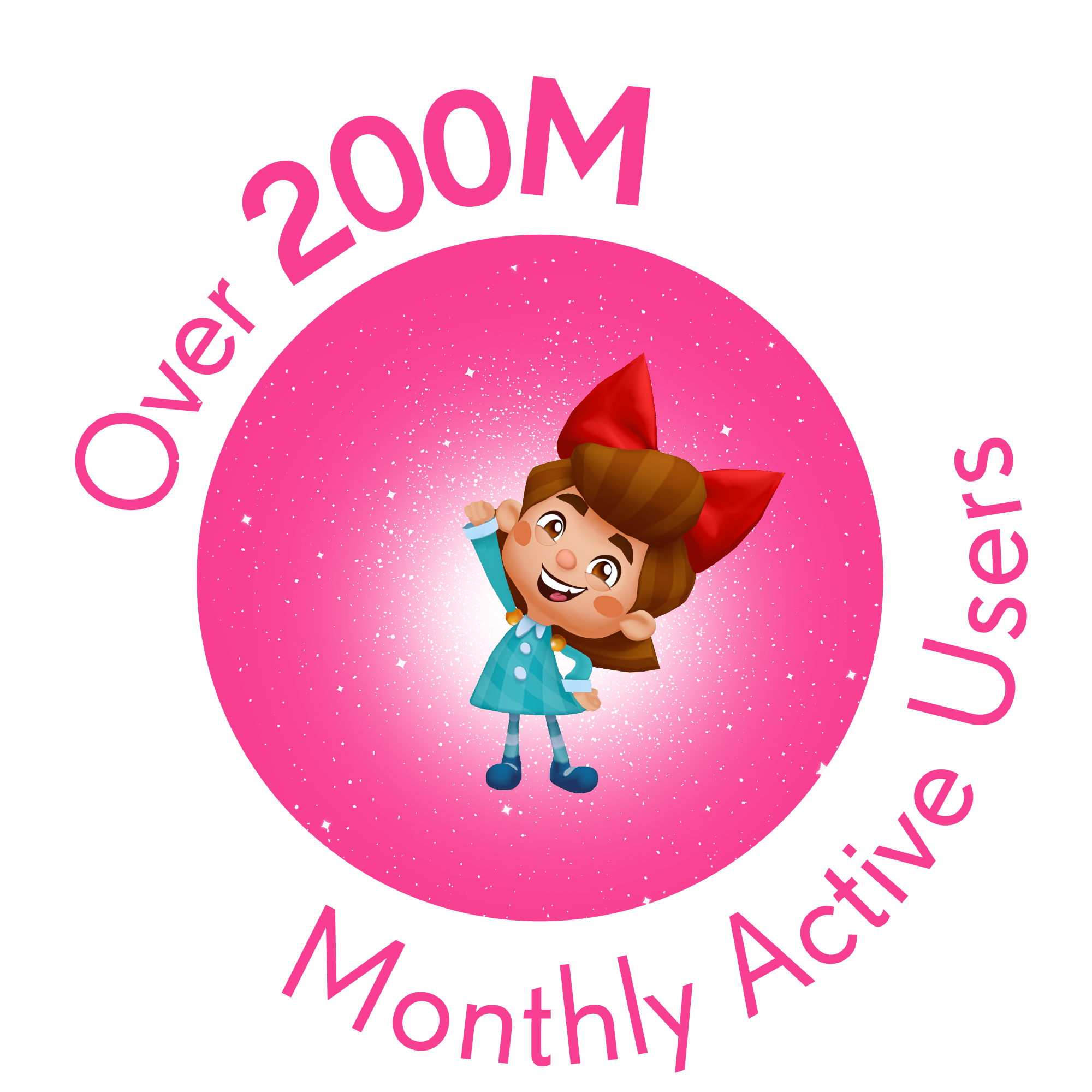
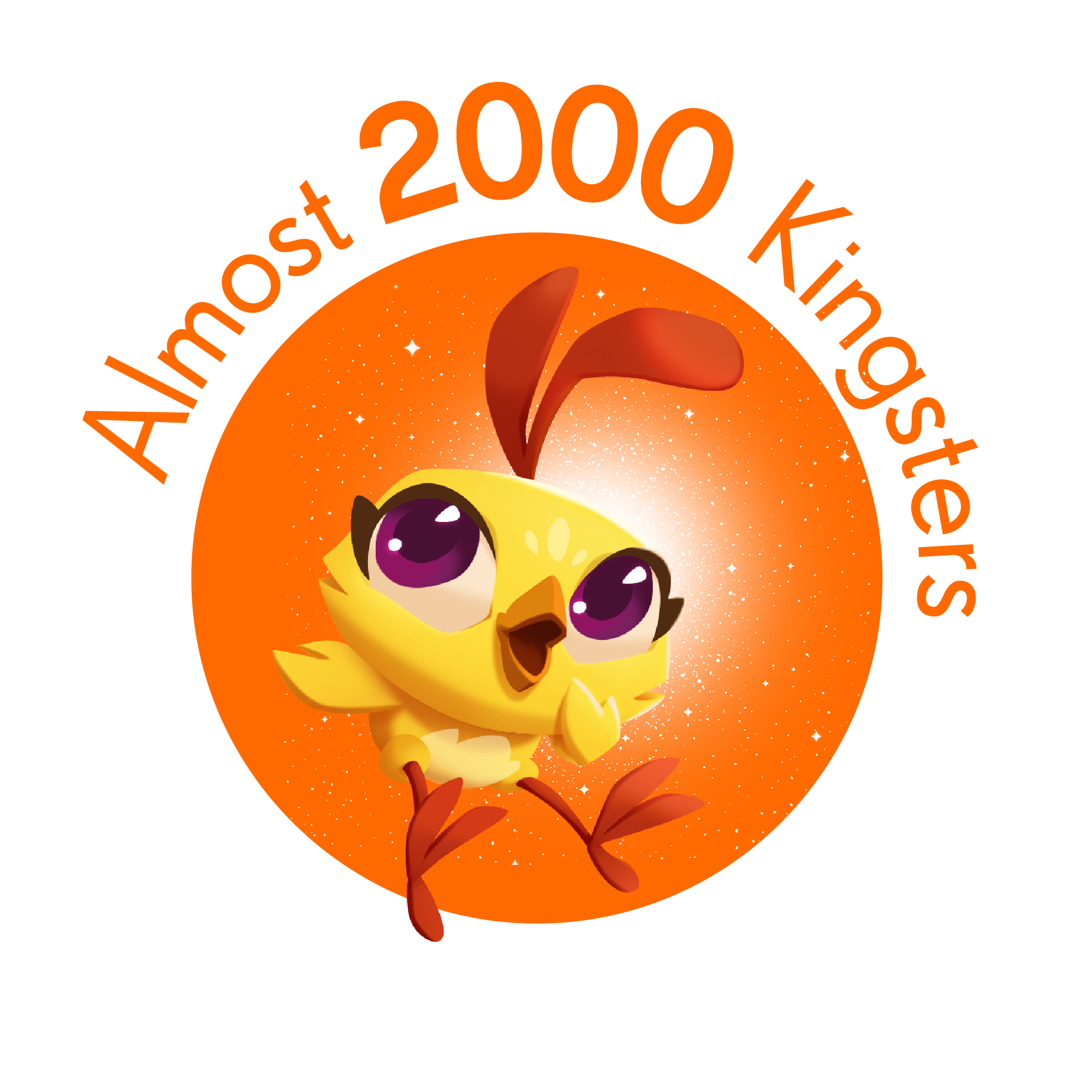
A sense of mutual respect and mindfulness permeates our culture-in fact, it’s the key to our success.
Our Crafts
A career at Phenom Foods Market is more than the work you do. impact on the community, your personal growth and team members.
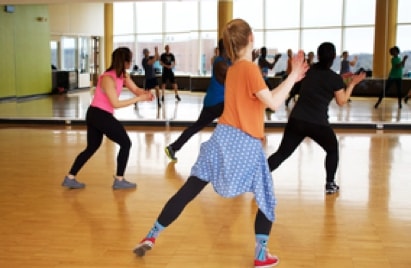
lorem ipsum
Games Design & Production
As level designers, economy designers, audio experts, project managers, and producers, bringing moments of joy to millions of people is all in a day’s work.
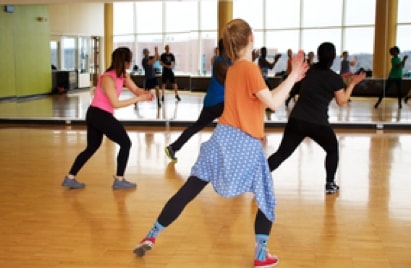
lorem ipsum
Technology & Development
We create unforgettable games that are loved by hundreds of millions of players around the world. Every day we experiment, prototype and challenge the norms.
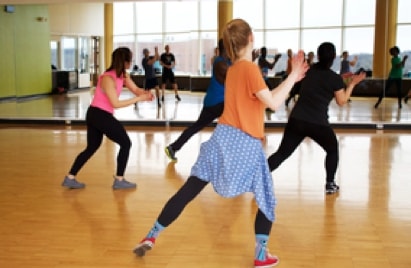
lorem ipsum
Art
Creators, imagineers, and visual storytellers. We bring beauty, excitement and depth to the games millions of people hold dear to their hearts.
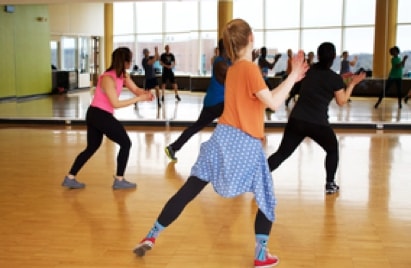
lorem ipsum
UX & UI
We are the Kingsters who look at the big picture, to uncover the small moments of joy we can bring into players’ lives.
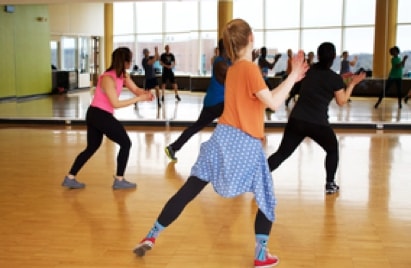
lorem ipsum
Data, Analytics & Strategy
We’re the magicians that inform King’s decisions. The data scientists who swap ‘boring’ for boring down into billions upon billions of gameplay events – every day – to deliver game-changing insights.
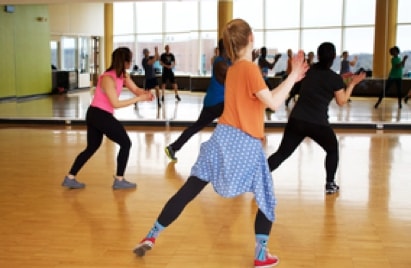
lorem ipsum
Customer & Game Support
We’re the Kingsters our players count on to resolve their issues, and the gatherers of insights that raise service levels across the Kingdom.
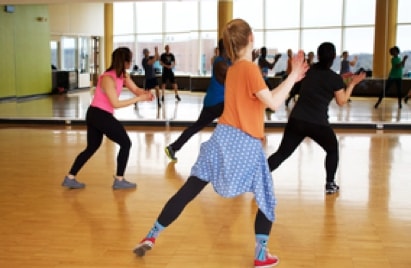
lorem ipsum
Marketing
We craft new ways to delight our community of players and create unforgettable events that inspire others to begin their quests.
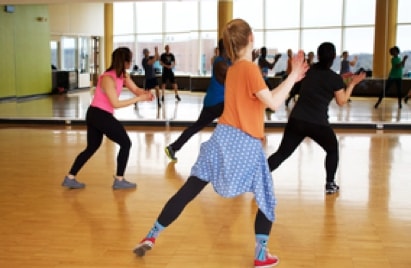
lorem ipsum
HR & Business Support
We craft the global culture that allows our fellow Kingsters to bring their whole selves to work. Our mission is to make the Kingdom a fun, friendly and playful place for everyone.
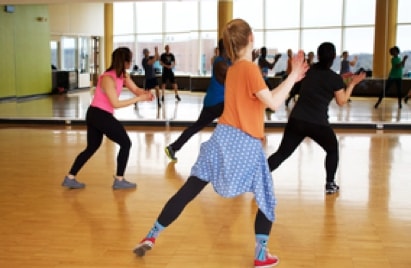
lorem ipsum
Finance
From business modelling to smart accounting, commercial insight to cross-team collaboration, we help the Kingdom continue to dream bigger.
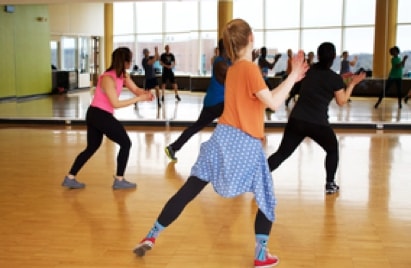
lorem ipsum
Legal
Change is constant so we’re always adapting at speed, advising our people and protecting the creativity of our teams.
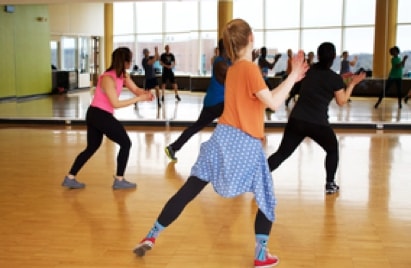
lorem ipsum
Advertising & Sales
We're shaping the industry and taking advertising to new levels.
A sense of mutual respect and mindfulness permeates our culture-in fact, it’s the key to our success.
Heading
There are many variations of passages of Lorem Ipsum available, but the majority have suffered alteration in some form, by injected humour, or randomised words which don't look even slightly believable.
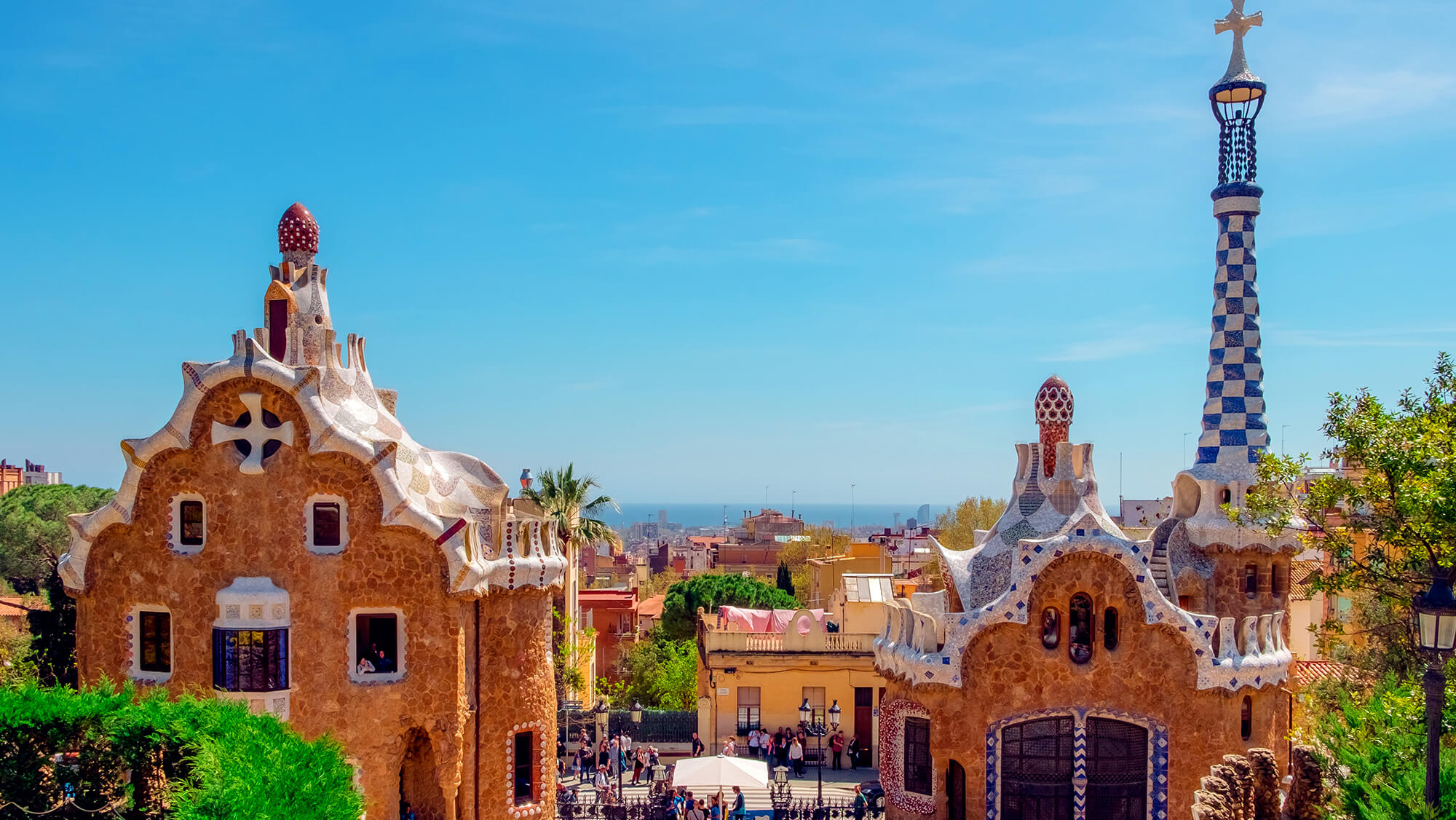
Our Locations
King has multiple locations around the world where our talented teams work to create amazing gaming experiences. From bustling cities to scenic landscapes, our workplaces are as diverse as the games we develop. Join us as we take you on a journey to some of our key locations.
A sense of mutual respect and mindfulness permeates our culture-in fact, it’s the key to our success.
Sound good? Join our Talent Community.
Lorem ipsum dolor sit amet consectetur adipisicing elit. Inventore assumenda asperiores distinctio est perferendis esse animi maiores dolor numquam nihil sint, et quae nobis harum consequuntur! Assumenda facere magnam nostrum?
A sense of mutual respect and mindfulness permeates our culture-in fact, it’s the key to our success.
#LifeAtKing
These are our six core values, and they guide and shape teh way we do business.
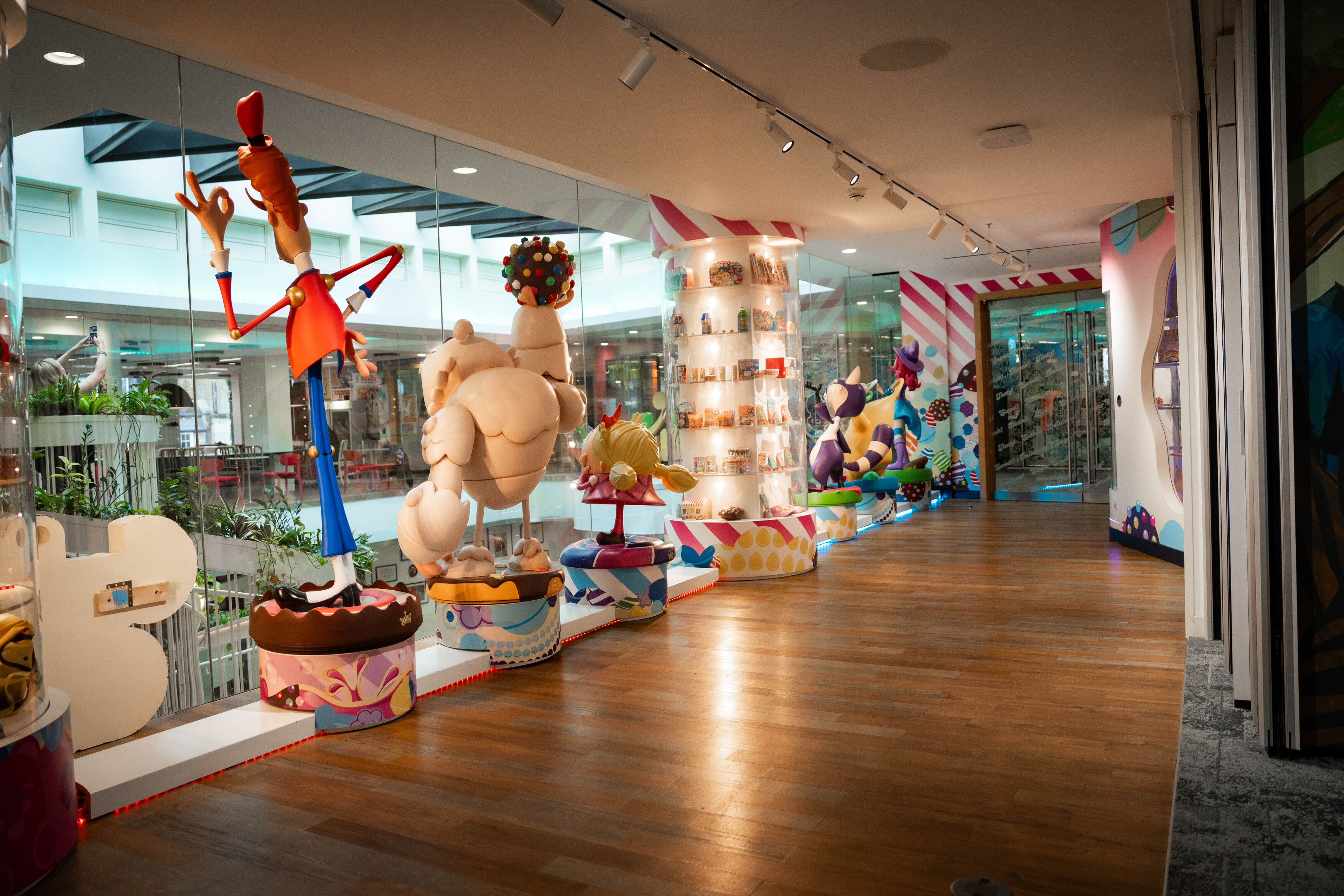
We Care About our Communities and Environment
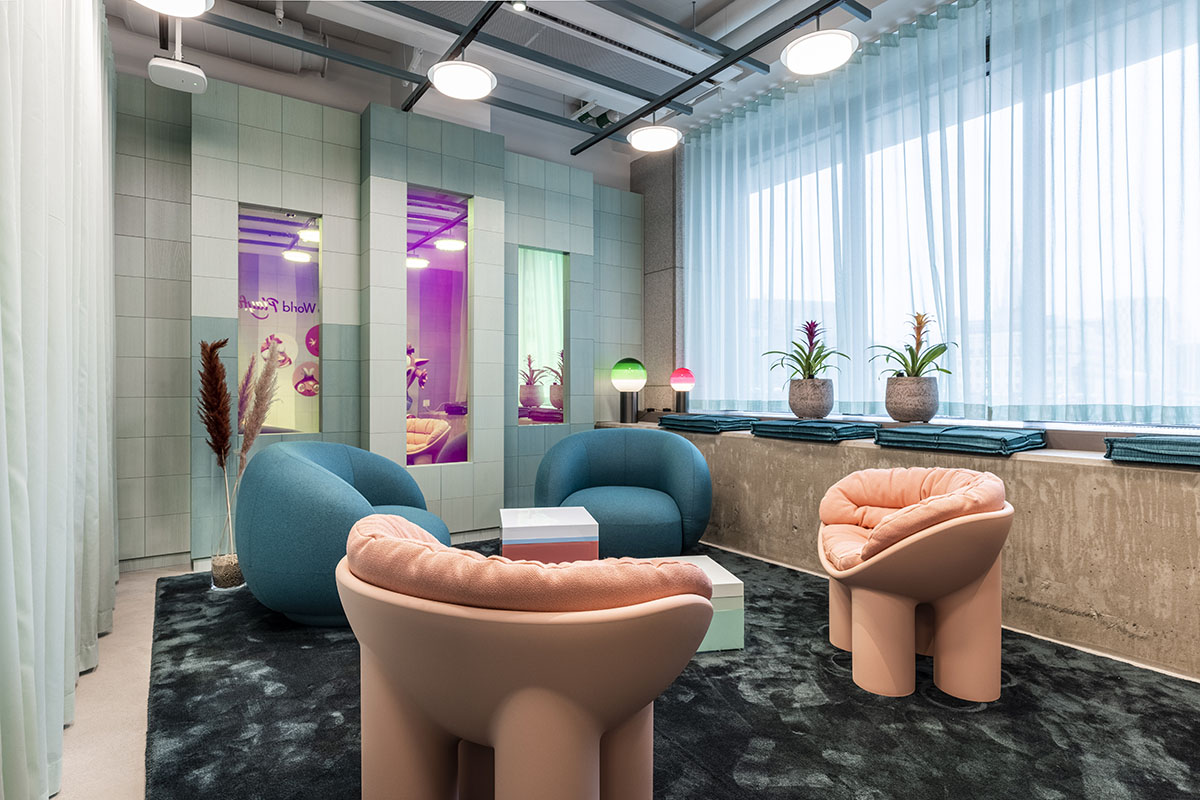
We Care About our Communities and Environment
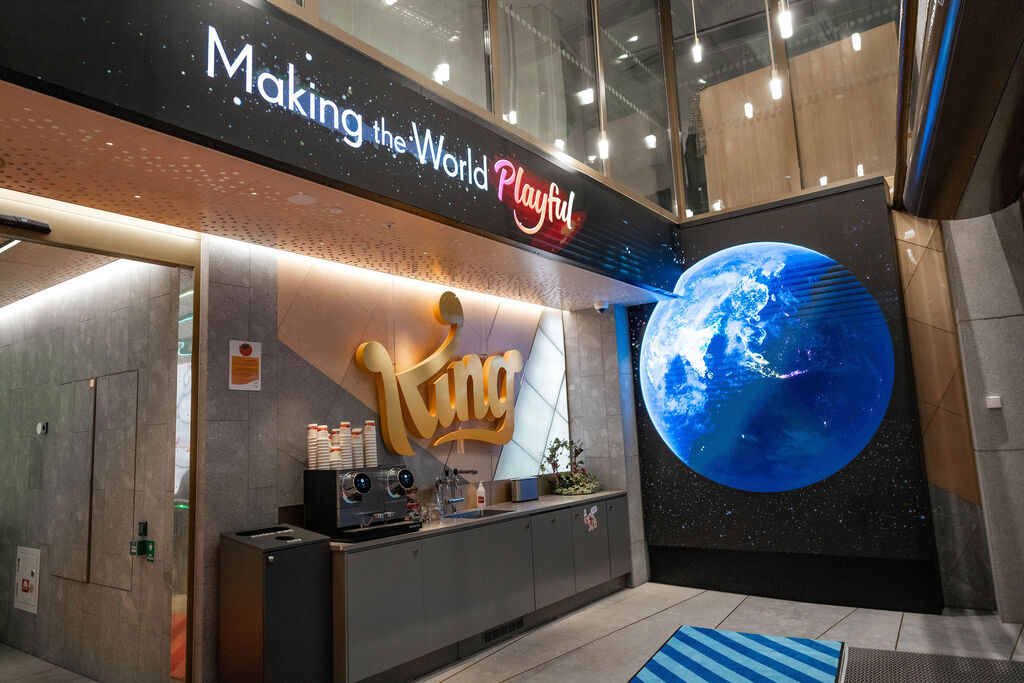
We Care About our Communities and Environment
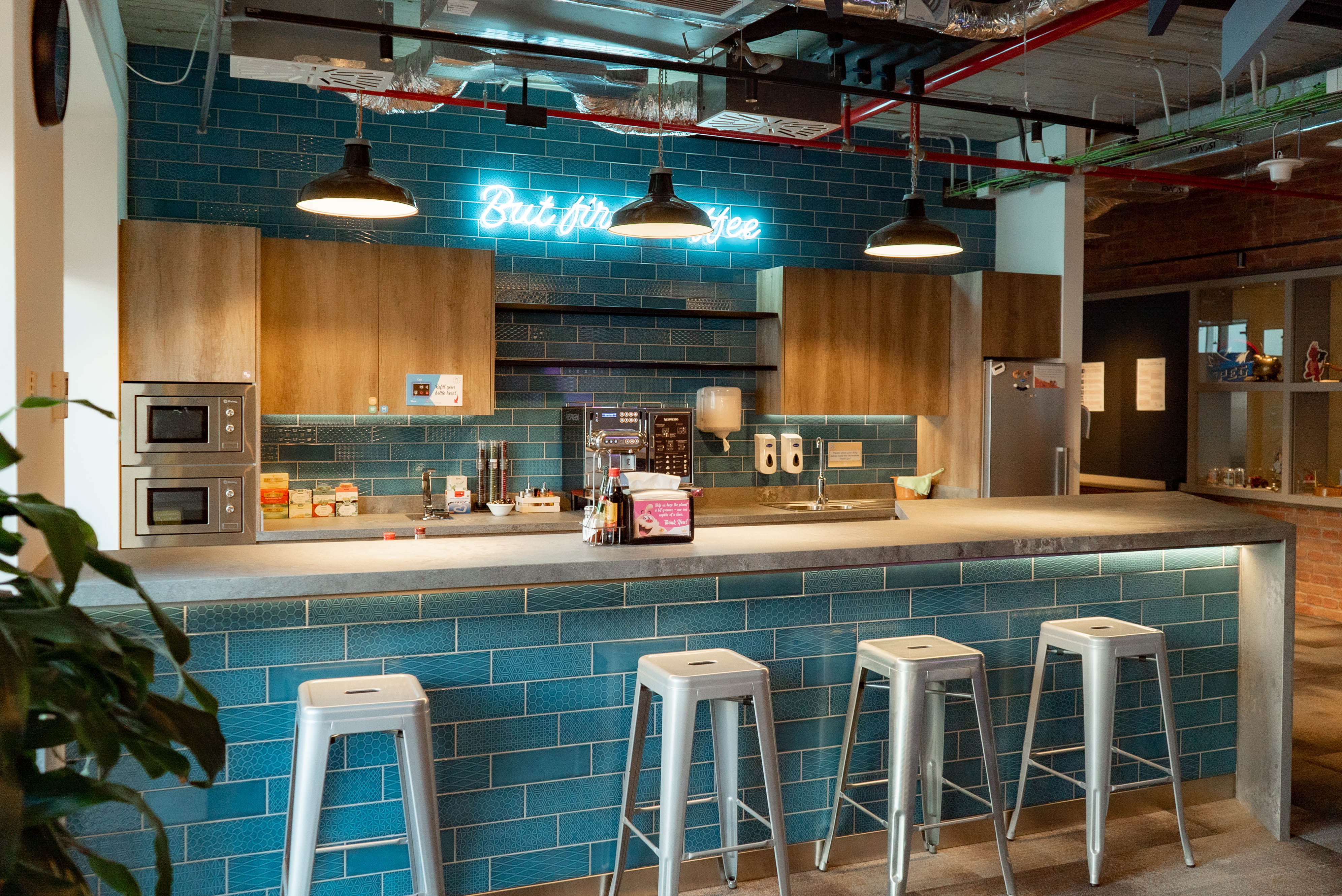
We Care About our Communities and Environment
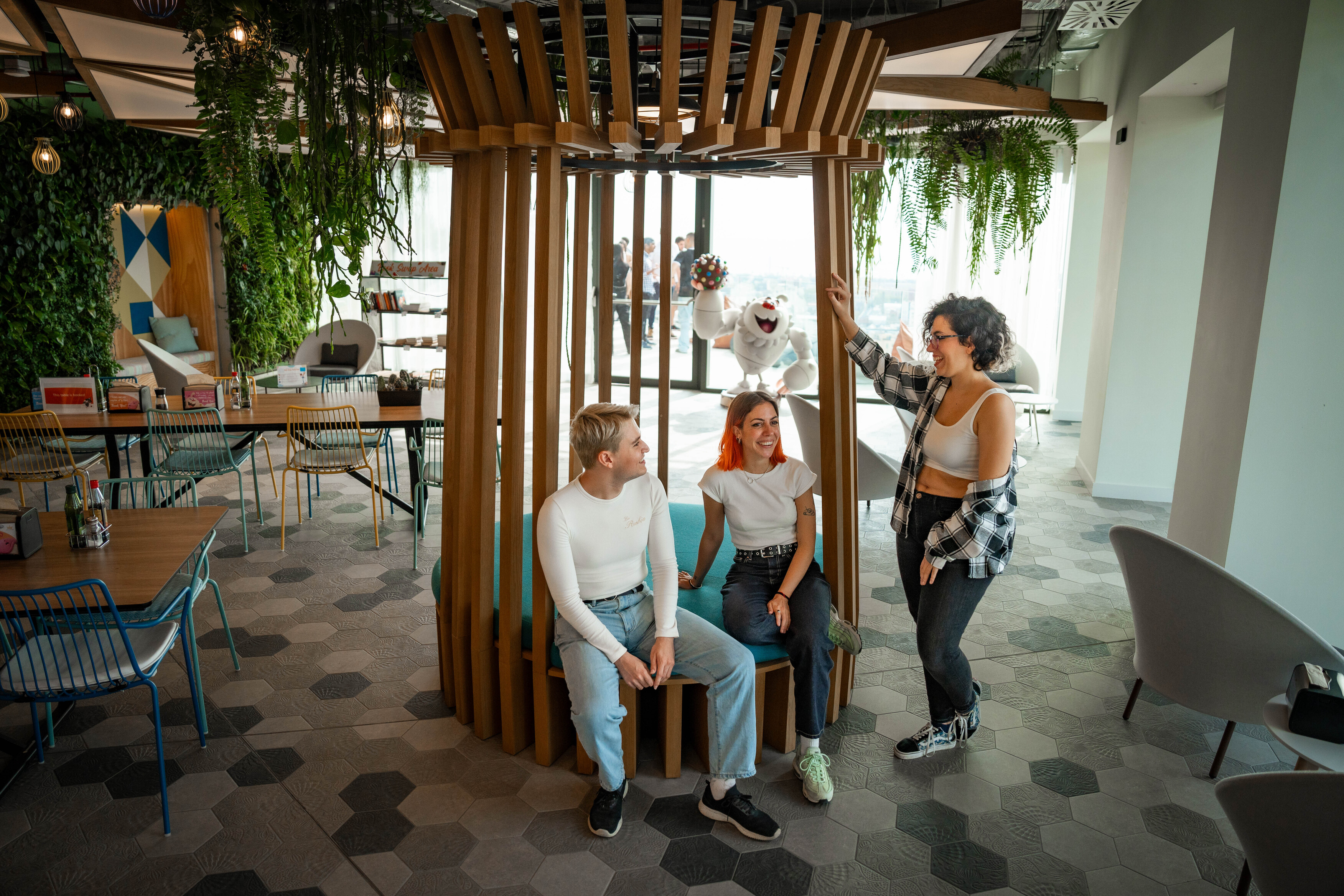
We Create Profits and Prosperity
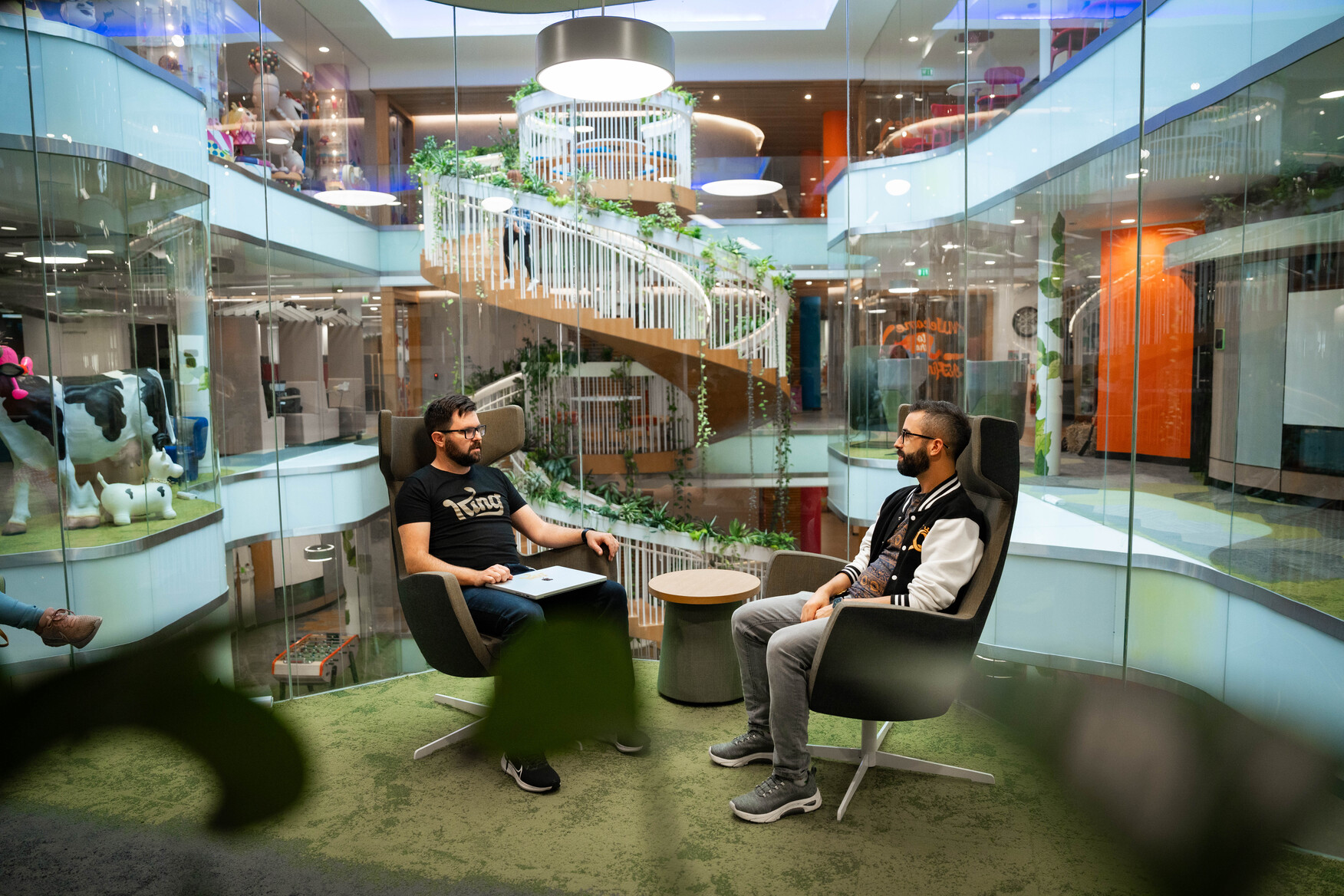
We Care About our Communities and Environment
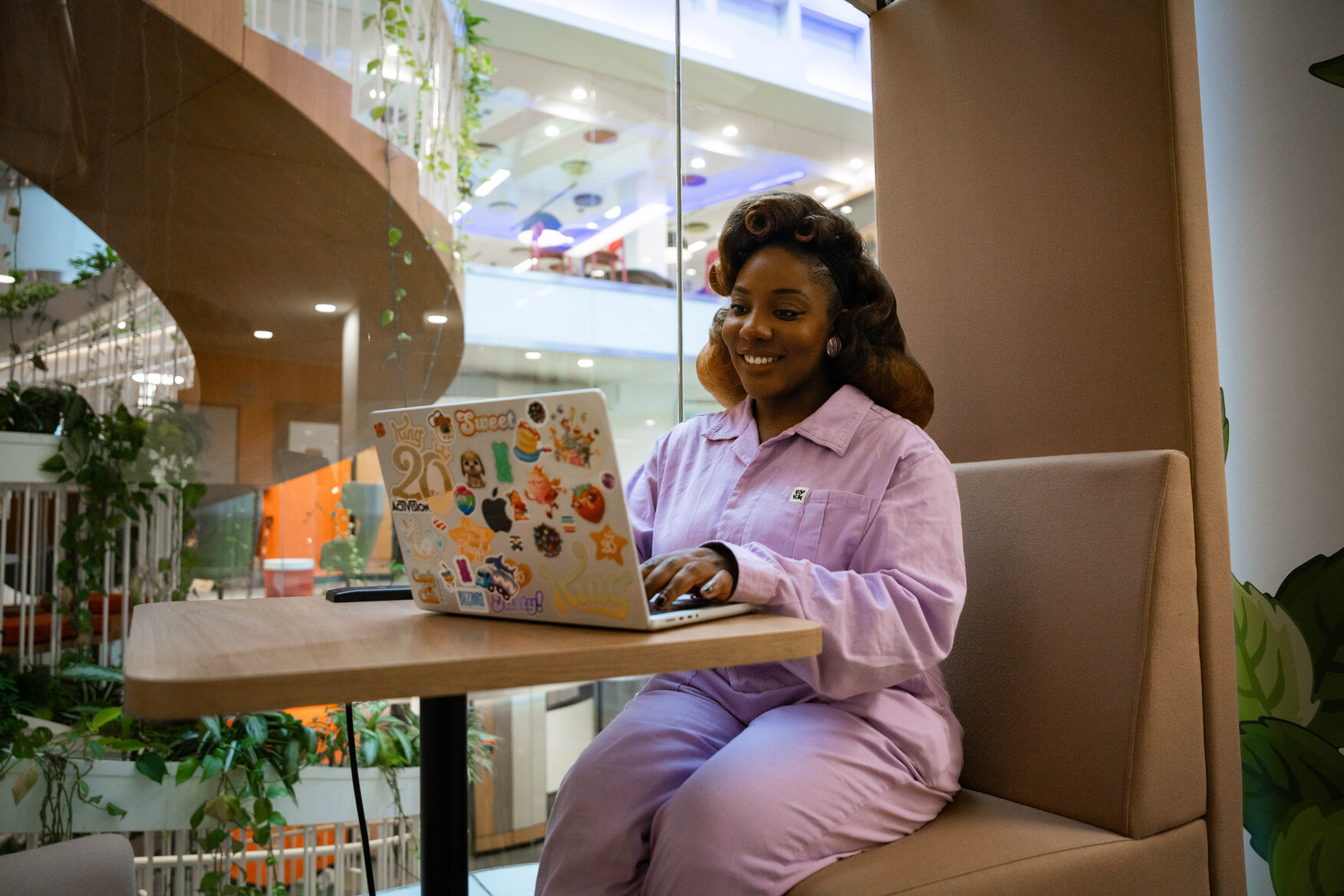
We Care About our Communities and Environment
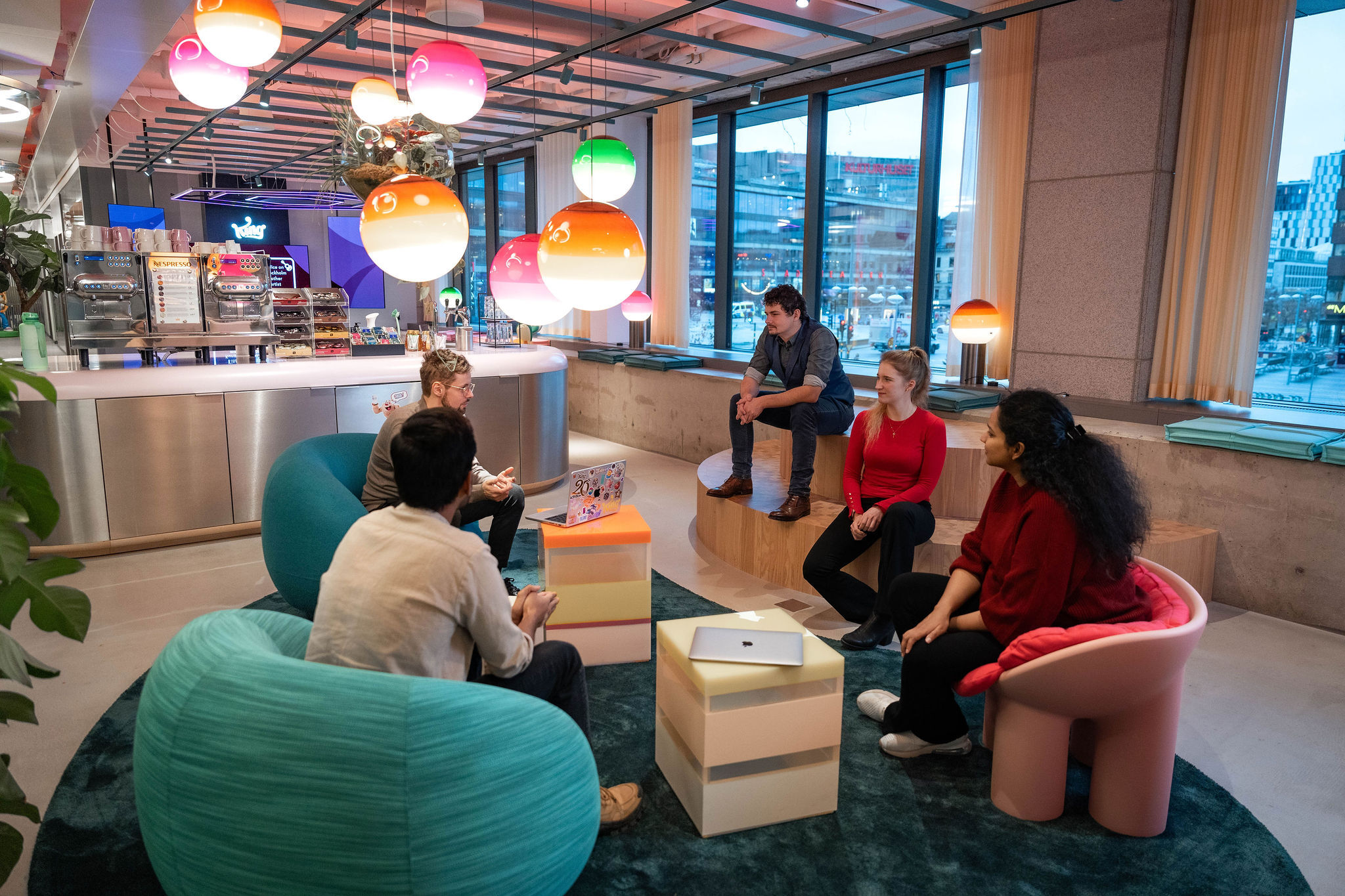
We Care About our Communities and Environment
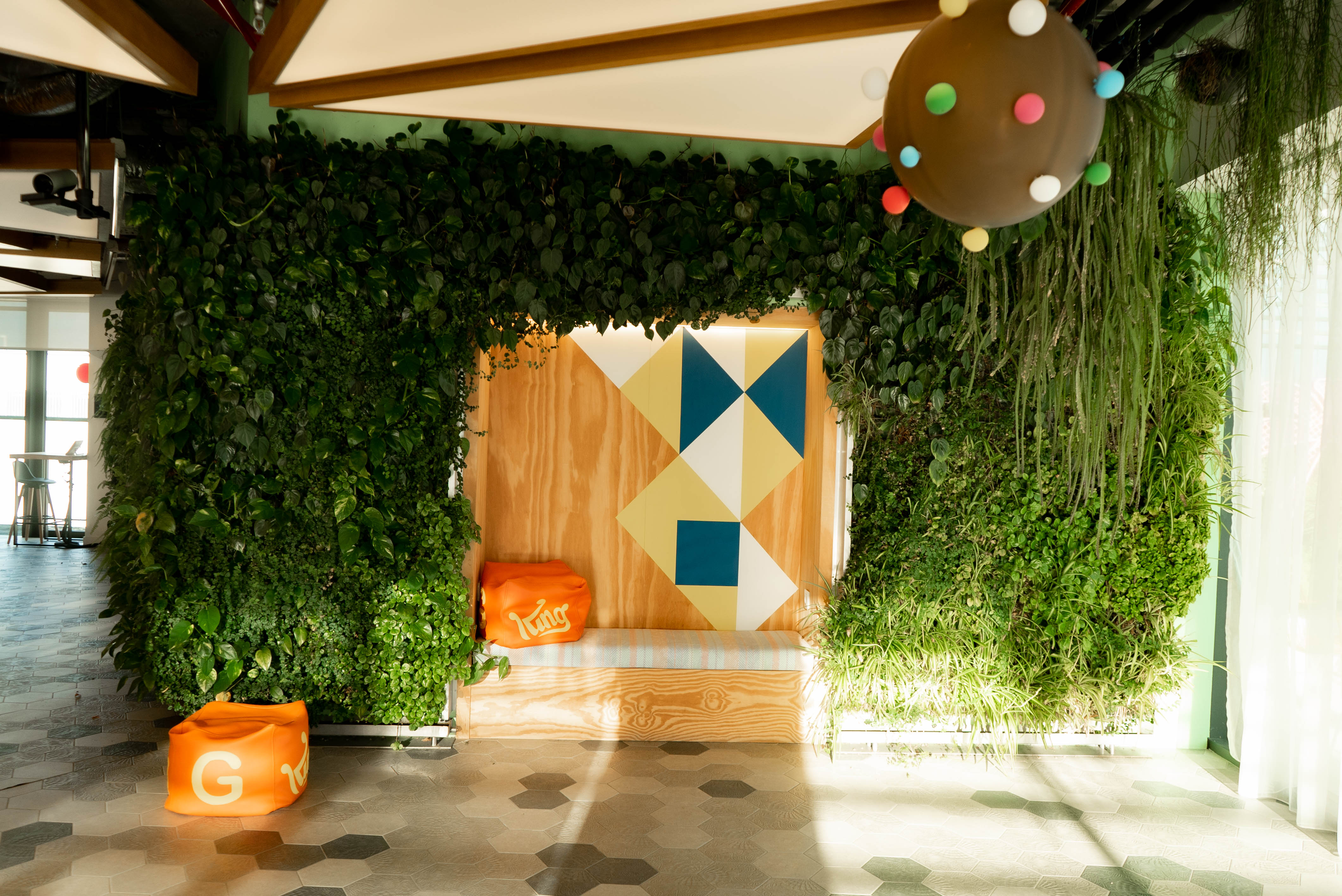
We Care About our Communities and Environment
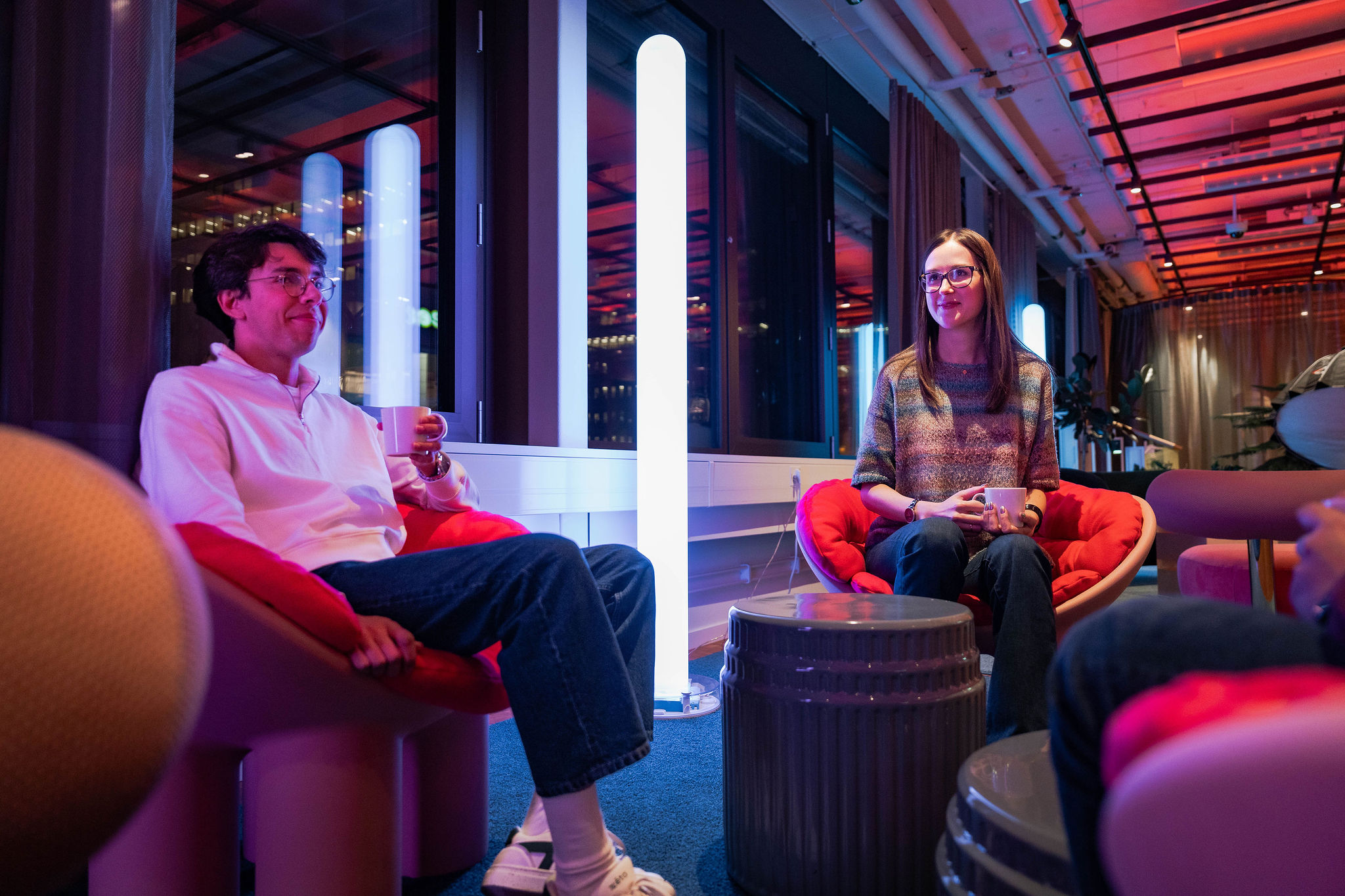
We Care About our Communities and Environment
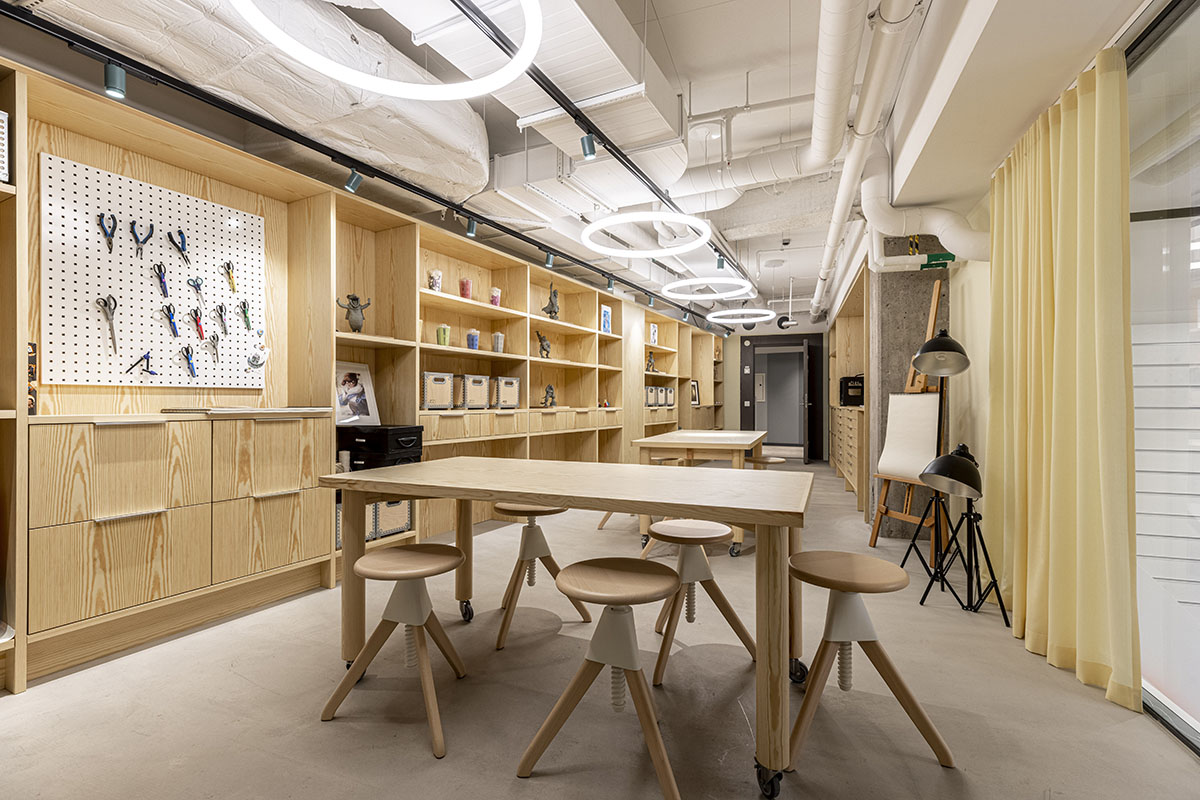
We Care About our Communities and Environment
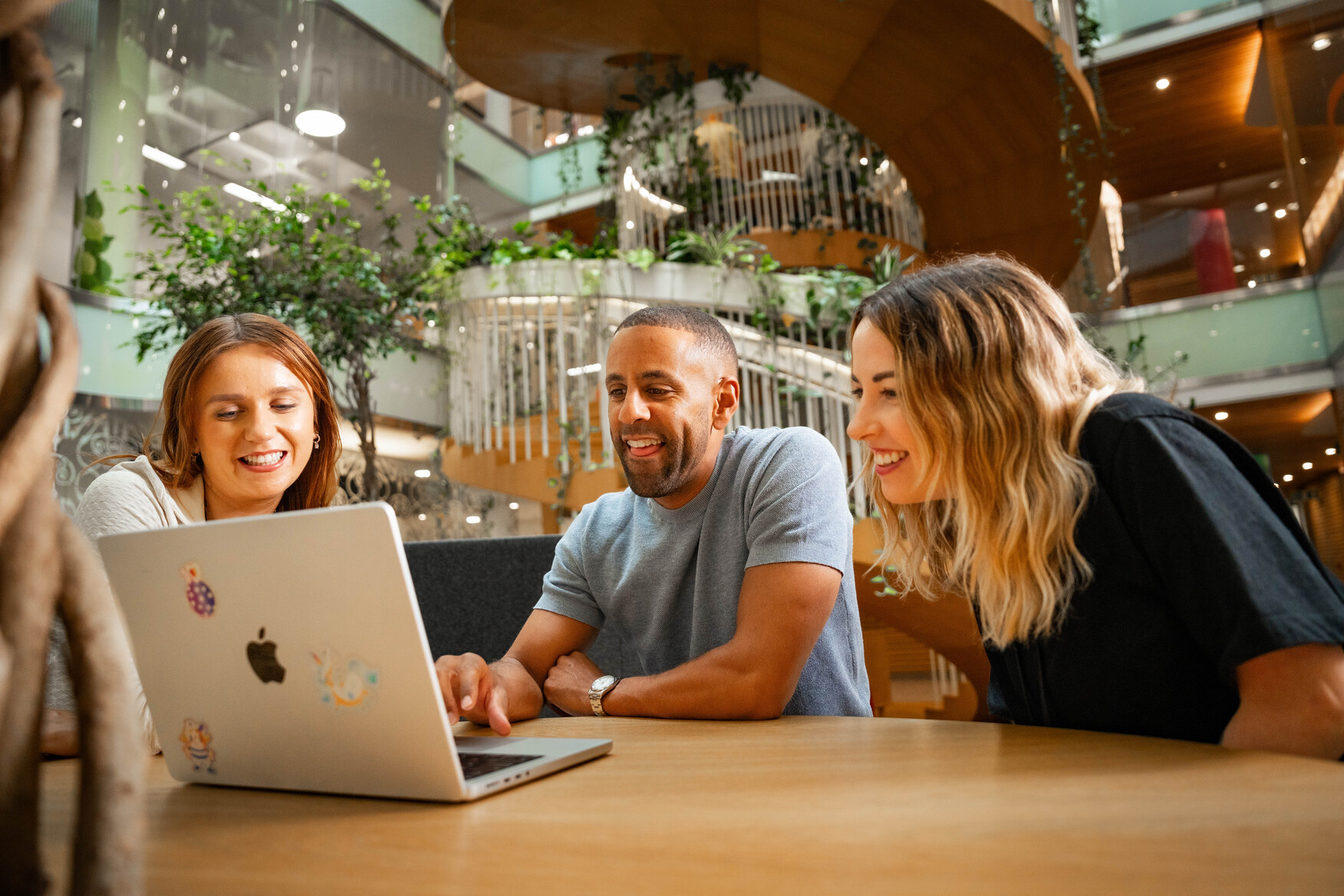
We Care About our Communities and Environment
A sense of mutual respect and mindfulness permeates our culture-in fact, it’s the key to our success.